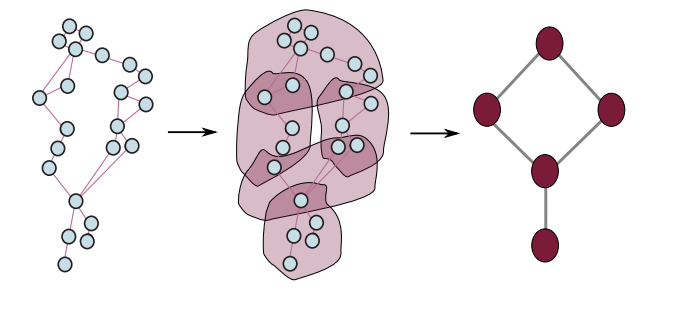
Position Paper: Challenges and Opportunities in Topological Deep Learning | ICML
Theodore Papamarkou, Tolga Birdal, Michael Bronstein, Gunnar Carlsson, Justin Curry, Yue Gao, Mustafa Hajij, Roland Kwitt, Pietro Liò, Paolo Di Lorenzo, Vasileios Maroulas, Nina Miolane, Farzana Nasrin, Karthikeyan Natesan Ramamurthy, Bastian Rieck, Simone Scardapane, Michael T. Schaub, Petar Veličković, Bei Wang, Yusu Wang, Guo-Wei Wei, Ghada Zamzmi
Topological deep learning (TDL) is a rapidly evolving field that uses topological features to understand and design deep learning models. This paper posits that TDL may complement graph representation learning and geometric deep learning by incorporating topological concepts, and can thus provide a natural choice for various machine learning settings. To this end, this paper discusses open problems in TDL, ranging from practical benefits to theoretical foundations. For each problem, it outlines potential solutions and future research opportunities. At the same time, this paper serves as an invitation to the scientific community to actively participate in TDL research to unlock the potential of this emerging field.
Read More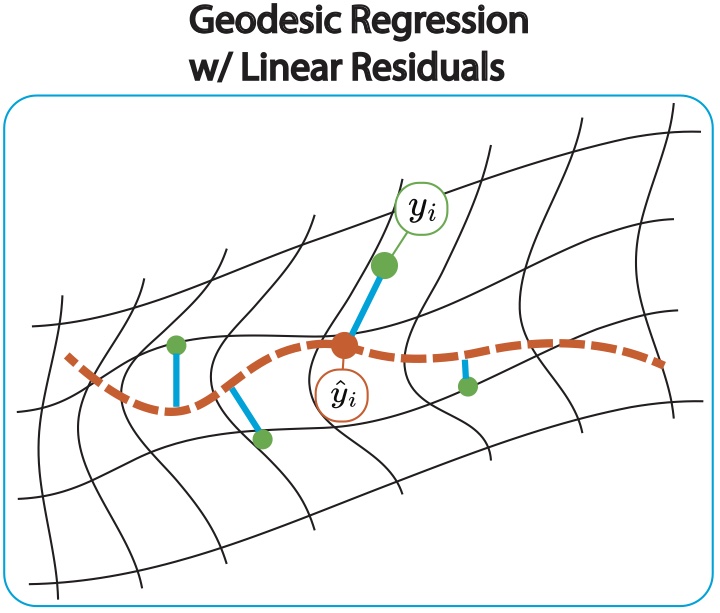
On Accuracy and Speed of Geodesic Regression: Do Geometric Priors Improve Learning on Small Datasets? | CVPRW L3D-IVU: Learning with Limited Labelled Data for Image and Video Understanding
Myers, A., Miolane, N.
Image datasets in specialized fields of science, such as biomedicine, are typically smaller than traditional machine learning datasets. As such, they present a problem for training many models. To address this challenge, researchers often attempt to incorporate priors, i.e., external knowledge, to help the learning procedure. Geometric priors, for example, offer to restrict the learning process to the manifold to which the data belong. However, learning on manifolds is sometimes computationally intensive to the point of being prohibitive. Here, we ask a provocative question: is machine learning on manifolds really more accurate than its linear counterpart to the extent that it is worth sacrificing significant speedup in computation? We answer this question through an extensive theoretical and experimental study of one of the most common learning methods for manifold-valued data: geodesic regression.
Read More
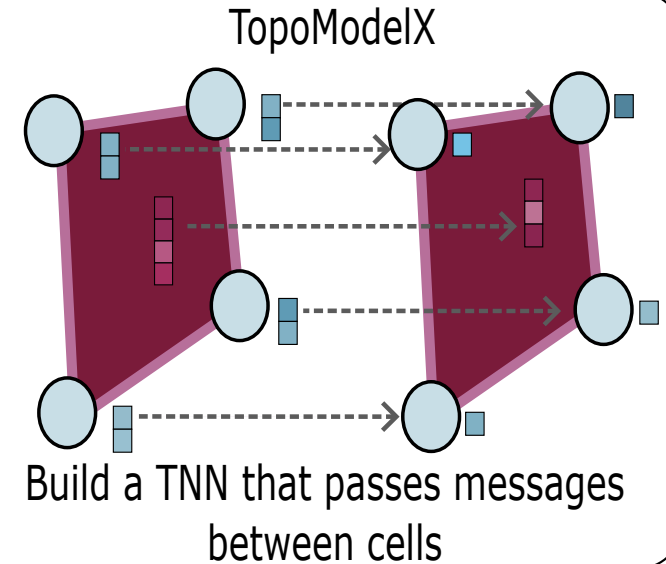
TopoX: A Suite of Python Packages for Machine Learning on Topological Domains | Submitted to the Journal of Machine Learning Research (JMLR)
Mustafa Hajij, Mathilde Papillon, Florian Frantzen, Jens Agerberg, Ibrahem AlJabea, Ruben Ballester, Claudio Battiloro, Guillermo Bernárdez, Tolga Birdal, Aiden Brent, Peter Chin, Sergio Escalera, Simone Fiorellino, Odin Hoff Gardaa, Gurusankar Gopalakrishnan, Devendra Govil, Josef Hoppe, Maneel Reddy Karri, Jude Khouja, Manuel Lecha, Neal Livesay, Jan Meißner, Soham Mukherjee, Alexander Nikitin, Theodore Papamarkou, Jaro Prílepok, Karthikeyan Natesan Ramamurthy, Paul Rosen, Aldo Guzmán-Sáenz, Alessandro Salatiello, Shreyas N. Samaga, Simone Scardapane, Michael T. Schaub, Luca Scofano, Indro Spinelli, Lev Telyatnikov, Quang Truong, Robin Walters, Maosheng Yang, Olga Zaghen, Ghada Zamzmi, Ali Zia, Nina Miolane
We introduce TopoX, a Python software suite that provides reliable and user-friendly building blocks for computing and machine learning on topological domains that extend graphs: hypergraphs, simplicial, cellular, path and combinatorial complexes. TopoX consists of three packages: TopoNetX facilitates constructing and computing on these domains, including working with nodes, edges and higher-order cells; TopoEmbedX provides methods to embed topological domains into vector spaces, akin to popular graph-based embedding algorithms such as node2vec; TopoModelx is built on top of PyTorch and offers a comprehensive toolbox of higher-order message passing functions for neural networks on topological domains. The extensively documented and unit-tested source code of TopoX is available under MIT license at this https URL.
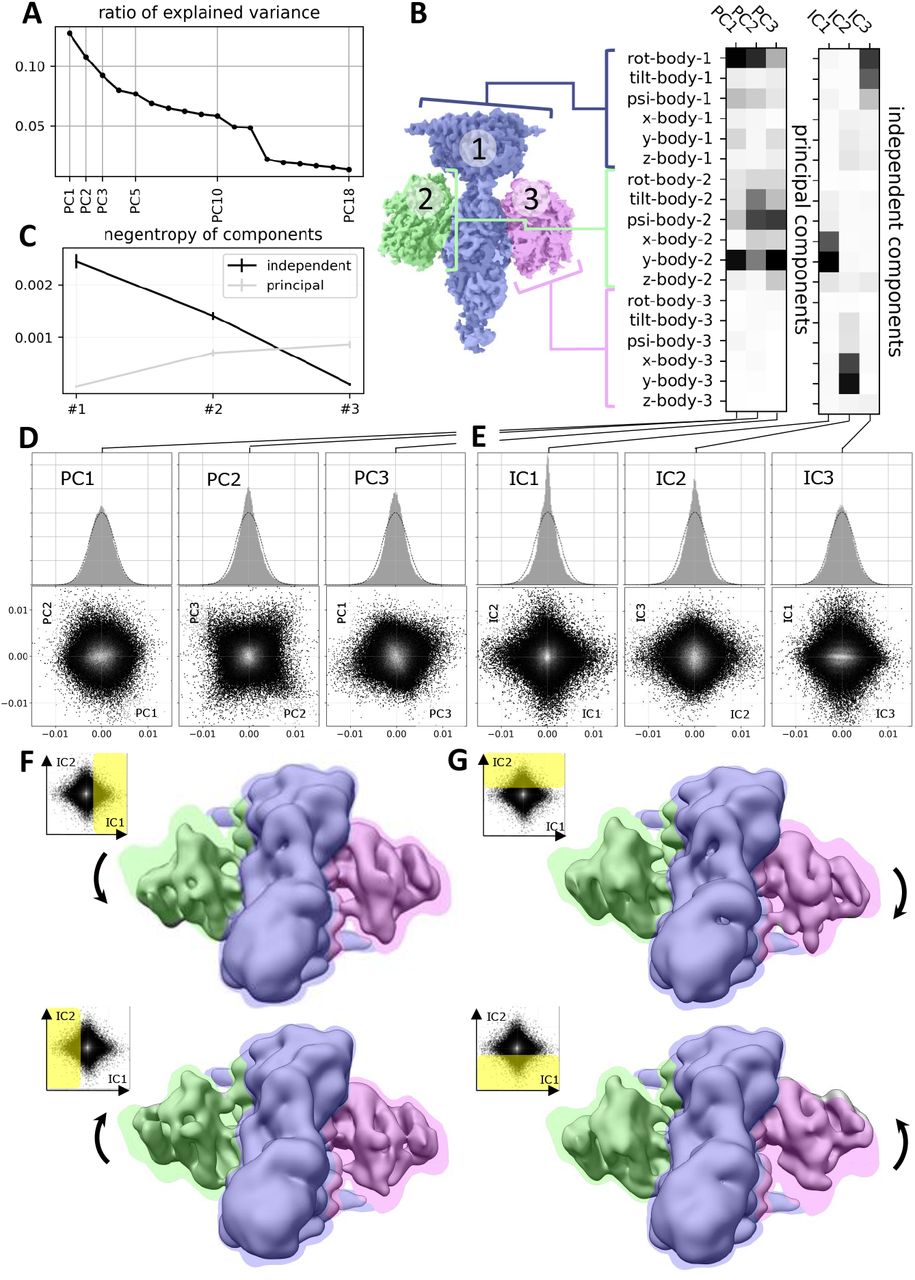
Towards Interpretable Cryo-EM: Disentangling Latent Spaces of Molecular Conformations | Submitted to Journal Frontiers in Molecular Biosciences
David A. Klindt, Aapo Hyvärinen, Axel Levy, Nina Miolane, Frédéric Poitevin
Molecules are essential building blocks of life and their different conformations (i.e., shapes) crucially determine the functional role that they play in living organisms. Cryogenic Electron Microscopy (cryo-EM) allows for acquisition of large image datasets of individual molecules. Recent advances in computational cryo-EM have made it possible to learn latent variable models of conformation landscapes. However, interpreting these latent spaces remains a challenge as their individual dimensions are often arbitrary. The key message of our work is that this interpretation challenge can be viewed as an Independent Component Analysis (ICA) problem where we seek models that have the property of identifiability. That means, they have an essentially unique solution, representing a conformational latent space that separates the different degrees of freedom a molecule is equipped with in nature. Thus, we aim to advance the computational field of cryo-EM beyond visualizations as we connect it with the theoretical framework of (nonlinear) ICA and discuss the need for identifiable models, improved metrics, and benchmarks. Moving forward, we propose future directions for enhancing the disentanglement of latent spaces in cryo-EM, refining evaluation metrics and exploring techniques that leverage physics-based decoders of biomolecular systems. Moreover, we discuss how future technological developments in time-resolved single particle imaging may enable the application of nonlinear ICA models that can discover the true conformation changes of molecules in nature. The pursuit of interpretable conformational latent spaces will empower researchers to unravel complex biological processes and facilitate targeted interventions. This has significant implications for drug discovery and structural biology more broadly. More generally, latent variable models are deployed widely across many scientific disciplines. Thus, the argument we present in this work has much broader applications in AI for science if we want to move from impressive nonlinear neural network models to mathematically grounded methods that can help us learn something new about nature.
Read More
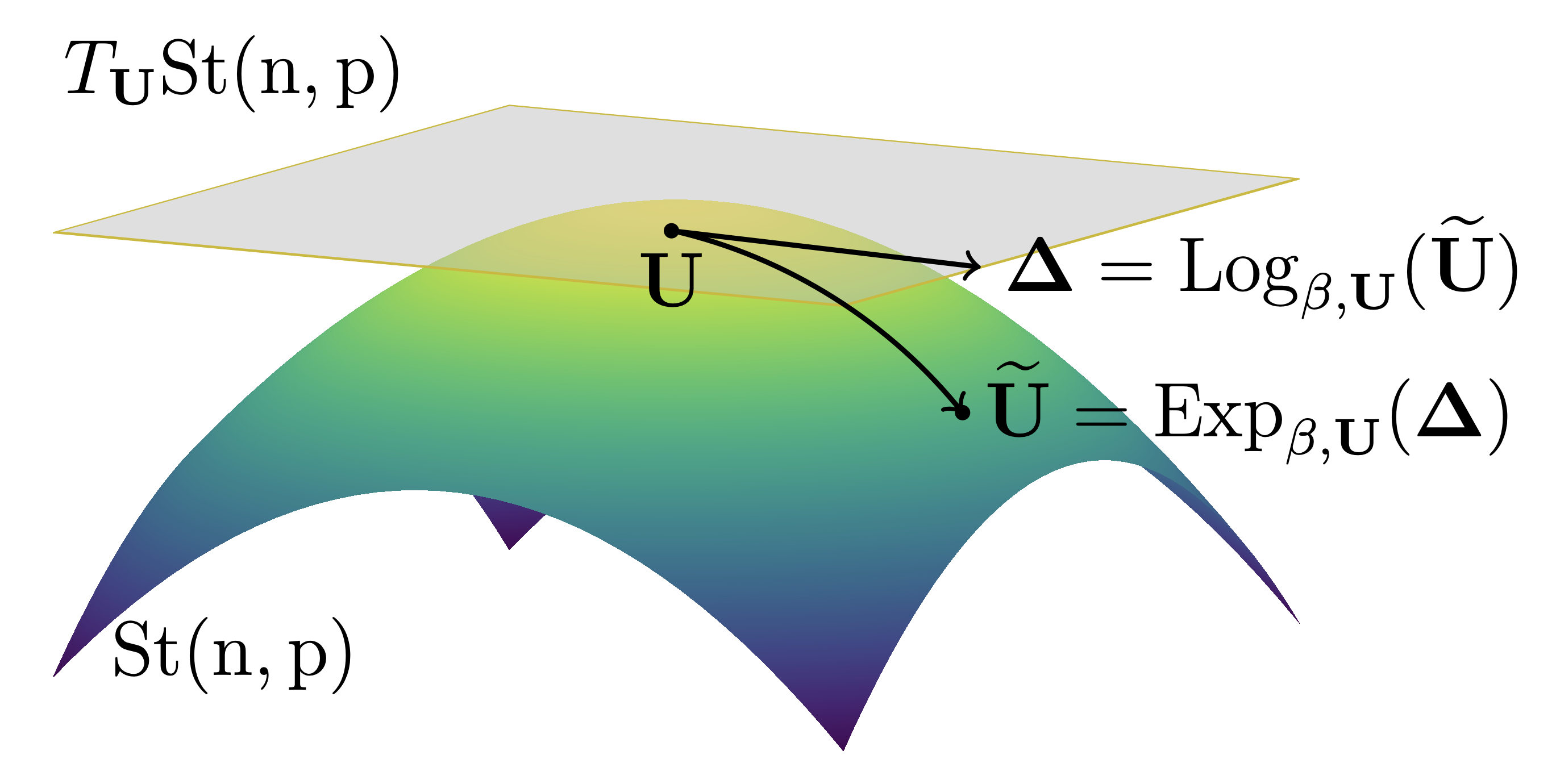
An efficient algorithm for the Riemannian logarithm on the Stiefel manifold for a family of Riemannian metrics | Submitted to SIAM Journal on Matrix Analysis and Applications (SIMAX)
Simon Mataigne, Ralf Zimmermann, and Nina Miolane
Since its popularization in 1998, the Stiefel manifold has proven crucial for addressing numerous issues in optimization, statistics, and machine learning. In 2021, Hüper and collaborators introduced a one-parameter family of Riemannian metrics on the Stiefel manifold, encompassing both the renowned Euclidean and canonical metrics. Zimmermann, in 2017, developed an especially effective technique to compute the Riemannian Log for the canonical metric, utilizing a pure matrix-algebraic method. Here, we present an extension of this approach applicable to the entire one-parameter family of Riemannian metrics.
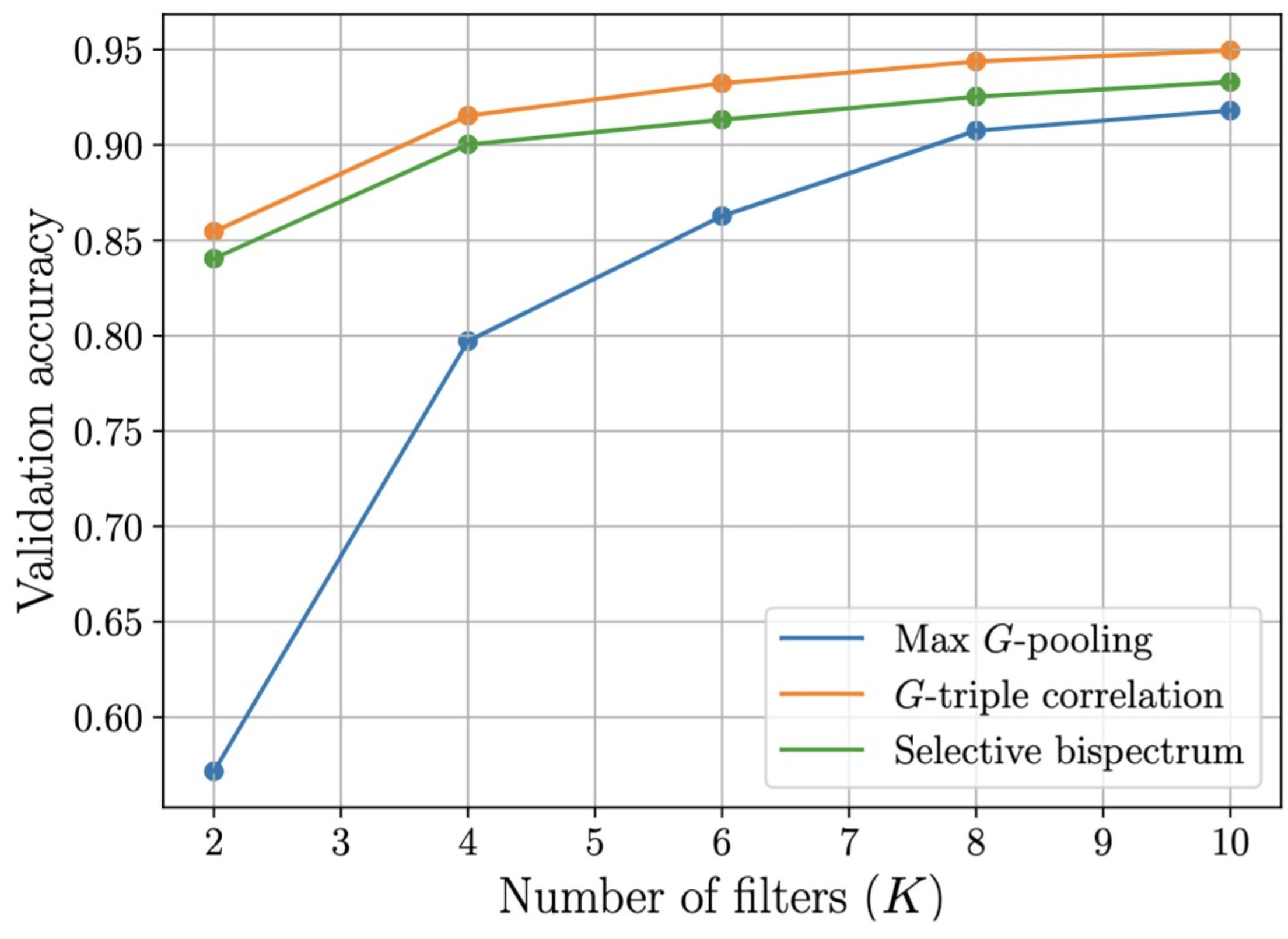
Efficient, Complete G-Invariance for G-Equivariant Networks via Algorithmic Reduction | Submitted to ICML
Simon Mataigne*, Johan Mathe*, Sophia Sanborn, Chris Hillar, Nina Miolane
G-CNNs extend CNNs' translation-equivariance to group-equivariance and invariance, leveraging symmetries like rotations. However, traditional group-invariance methods can be overly invariant, reducing robustness to adversarial attacks. The G-triple correlation (G-TC) method improves invariance and robustness but increases computational costs. This paper presents the selective G-bispectrum, a novel invariant layer using the Fourier transform of G-TC, but providing novel mathematical properties that reduce its computational load while preserving G-TC's benefits. Theoretical and experimental findings show that selective G-bispectrum maintains selective invariance with fewer coefficients offering a cost-effective alternative.
Read More
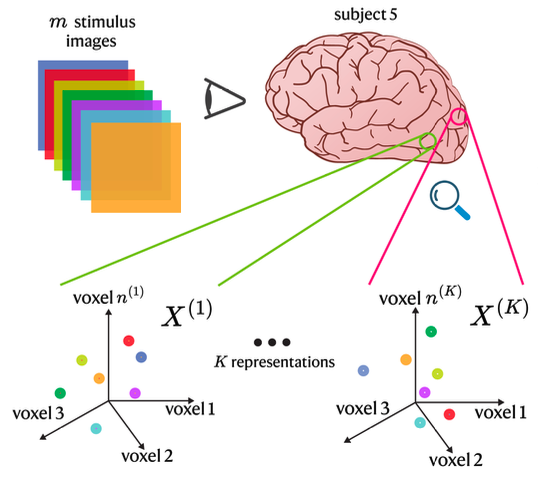
Relating Representational Geometry to Cortical Geometry in the Visual Cortex | NeurIPS Workshop on Unifying Representations in Neural Models
Francisco Acosta, Colin Conwell, Sophia Sanborn, David Klindt, Nina Miolane
A fundamental principle of neural representation is to minimize wiring length by spatially organizing neurons according to the frequency of their communication [Sterling and Laughlin, 2015]. A consequence is that nearby regions of the brain tend to represent similar content. This has been explored in the context of the visual cortex in recent works [Doshi and Konkle, 2023, Tong et al., 2023]. Here, we use the notion of cortical distance as a baseline to ground, evaluate, and interpret mea- sures of representational distance. We compare several popular methods—both second-order methods (Representational Similarity Analysis, Centered Kernel Alignment) and first-order methods (Shape Metrics)—and calculate how well the representational distance reflects 2D anatomical distance along the visual cortex (the anatomical stress score). We evaluate these metrics on a large-scale fMRI dataset of human ventral visual cortex [Allen et al., 2022b], and observe that the 3 types of Shape Metrics produce representational-anatomical stress scores with the smallest variance across subjects, (Z score = -1.5), which suggests that first-order representational scores quantify the relationship between representational and cortical geometry in a way that is more invariant across different subjects. Our work establishes a criterion with which to compare methods for quantifying representational similarity with implications for studying the anatomical organization of high-level ventral visual cortex.
Read More
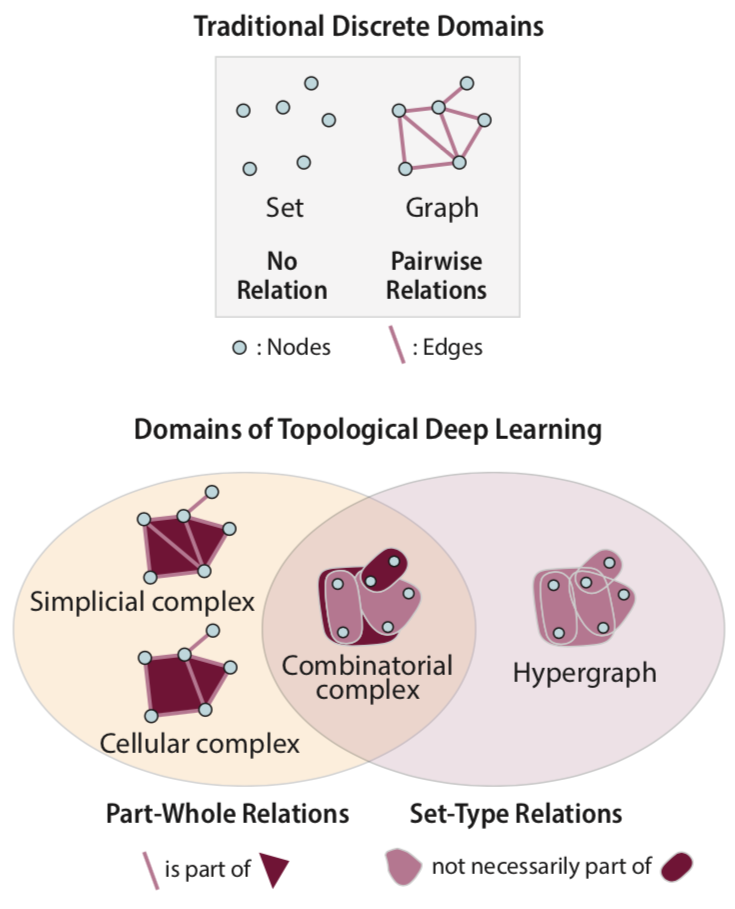
ICML 2023 Topological Deep Learning Challenge: Design and Results | PMLR
Papillon, M., Miolane, N., et al.
This paper presents the computational challenge on topological deep learning that was hosted within the ICML 2023 Workshop on Topology and Geometry in Machine Learning. The competition asked participants to provide open-source implementations of topological neural networks from the literature by contributing to the python packages TopoNetX (data processing) and TopoModelX (deep learning). The challenge attracted twenty-eight qualifying submissions in its two month duration. This paper describes the design of the challenge and summarizes its main findings.
Read More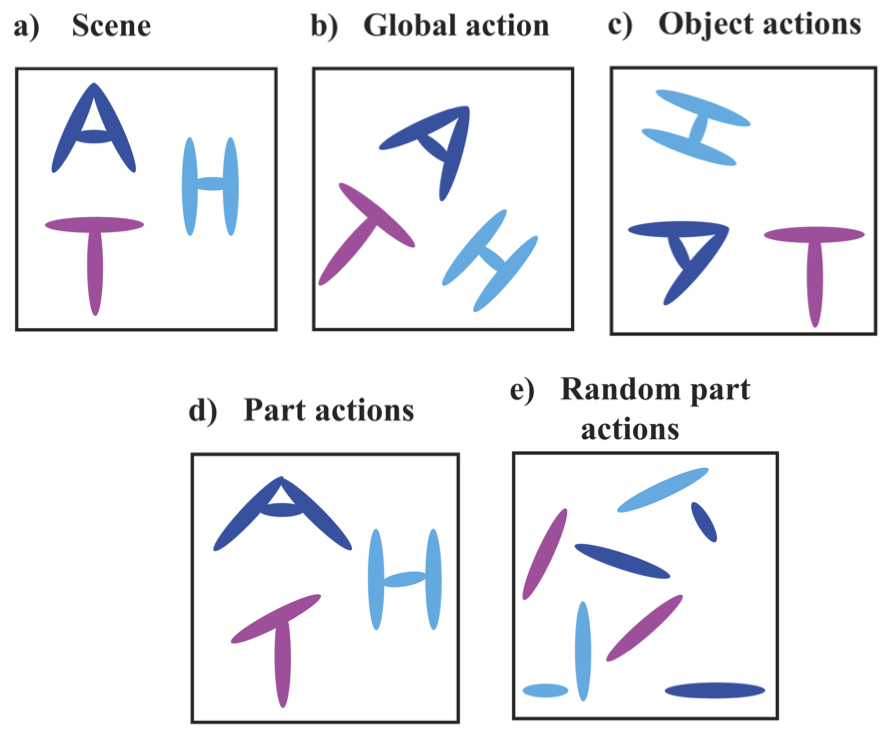
Visual Scene Representation with Hierarchical Equivariant Sparse Coding | PMLR
Shewmake, C., Buracas, D., Lillemark, H., Shin, J., Bekkers, E., Miolane, N., Olshausen, B.
We propose a hierarchical neural network architecture for unsupervised learning of equivariant part-whole decompositions of visual scenes. In contrast to the global equivariance of group-equivariant networks, the proposed architecture exhibits equivariance to part-whole transformations throughout the hierarchy, which we term hierarchical equivariance. The model achieves these structured internal representations via hierarchical Bayesian inference, which gives rise to rich bottom-up, top-down, and lateral information flows, hypothesized to underlie the mechanisms of perceptual inference in visual cortex. We demonstrate these useful properties of the model on a simple dataset of scenes with multiple objects under independent rotations and translations.
Read More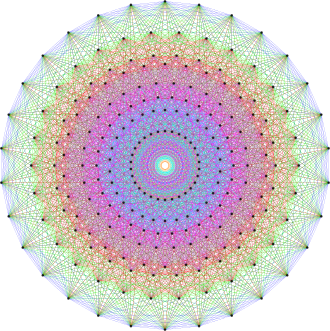
Preface: NeurIPS Workshop on Symmetry and Geometry in Neural Representations | PMLR
Sanborn, S., Shewmake, C., Azeglio, S., Di Bernardo, A., Miolane, N.
The first annual NeurIPS Workshop on Symmetry and Geometry in Neural Representations (NeurReps) was conceived to bring together researchers at the nexus of applied geometry, deep learning, and neuroscience, with the goal of advancing this understanding and illuminating geometric principles for neural information processing. Ultimately, we hope that this venue and associated community will support the development of the geometric approach to understanding neural representations, while strengthening ties to the mathematics community. The Neural Information Processing Systems (NeurIPS) conference historically emerged from the field of theoretical neuroscience or “connectionism.” This venue thus further serves the workshop’s goal of reinforcing the bond between deep learning and neuroscience.
Read More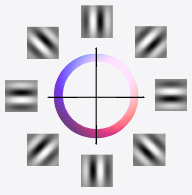
A General Framework for Robust G-Invariance in G-Equivariant Networks | NeurIPS
Sanborn, S., Miolane, N.
We introduce a general method for achieving robust group-invariance in group-equivariant convolutional neural networks (G-CNNs), which we call the G-triple-correlation (G-TC) layer. The approach leverages the theory of the triple-correlation on groups, which is the unique, lowest-degree polynomial invariant map that is also complete. Many commonly used invariant maps - such as the max - are incomplete: they remove both group and signal structure. A complete invariant, by contrast, removes only the variation due to the actions of the group, while preserving all information about the structure of the signal.
Read More
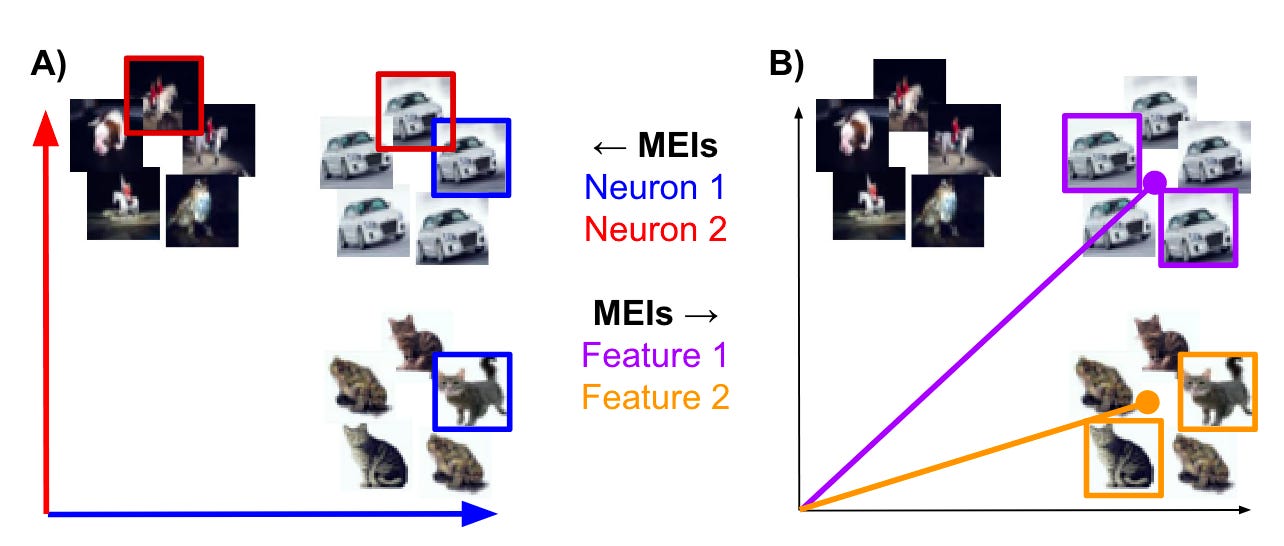
Identifying Interpretable Visual Features in Artifical and Biological Neural Systems | arXiv
Klindt, D., Sanborn, S., Acosta, F., Poitevin, F., Miolane, N.
Single neurons in neural networks are often interpretable in that they represent individual, intuitively meaningful features. However, many neurons exhibit mixed selectivity, i.e., they represent multiple unrelated features. A recent hypothesis proposes that features in deep networks may be represented in superposition, i.e., on non-orthogonal axes by multiple neurons, since the number of possible interpretable features in natural data is generally larger than the number of neurons in a given network. Accordingly, we should be able to find meaningful directions in activation space that are not aligned with individual neurons. Here, we propose (1) an automated method for quantifying visual interpretability that is validated against a large database of human psychophysics judgments of neuron interpretability, and (2) an approach for finding meaningful directions in network activation space. We leverage these methods to discover directions in convolutional neural networks that are more intuitively meaningful than individual neurons, as we confirm and investigate in a series of analyses. Moreover, we apply the same method to three recent datasets of visual neural responses in the brain and find that our conclusions largely transfer to real neural data, suggesting that superposition might be deployed by the brain. This also provides a link with disentanglement and raises fundamental questions about robust, efficient and factorized representations in both artificial and biological neural systems.
Read More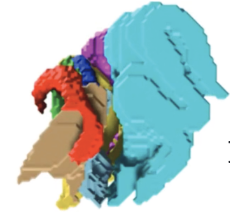
Geodesic Regression Characterizes 3D Shape Changes in the Female Brain During Menstruation | ICCV Computer Vision for Automated Medical Diagnosis
Myers, A., Taylor, C., Jacobs, E., Miolane, N. [Code]
Women are at higher risk of Alzheimer's and other neurological diseases after menopause, and yet research connecting female brain health to sex hormone fluctuations is limited. We seek to investigate this connection by developing tools that quantify 3D shape changes that occur in the brain during sex hormone fluctuations. Geodesic regression on the space of 3D discrete surfaces offers a principled way to characterize the evolution of a brain's shape. However, in its current form, this approach is too computationally expensive for practical use. In this paper, we propose approximation schemes that accelerate geodesic regression on shape spaces of 3D discrete surfaces. We also provide rules of thumb for when each approximation can be used. We test our approach on synthetic data to quantify the speed-accuracy trade-off of these approximations and show that practitioners can expect very significant speed-up while only sacrificing little accuracy. Finally, we apply the method to real brain shape data and produce the first characterization of how the female hippocampus changes shape during the menstrual cycle as a function of progesterone: a characterization made (practically) possible by our approximation schemes. Our work paves the way for comprehensive, practical shape analyses in the fields of bio-medicine and computer vision.
Read More
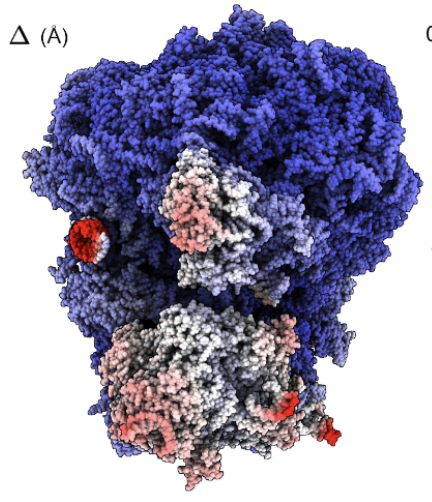
Unsupervised learning of structural variability in cryo-EM data using normal mode analysis of deformable atomic models. | Submitted to Nature Methods
Nashed, Y., Martel, J., Peck, A., Levy, A., Wetzstein, G., Miolane, N., Ratner, D., Poitevin, F.
Cryogenic electron microscopy (cryo-EM) has emerged as the method of choice to characterize the
structural variability of biomolecules at near-atomic resolution. We present a reconstruction approach
that eliminates the need for post-hoc atomic model fitting in 3D maps by deforming a given atomic
model along its normal modes directly against the 2D data. This end-to-end approach inherently
reduces the risk of error propagation while increasing interpretability of resulting structural ensembles.
Read More
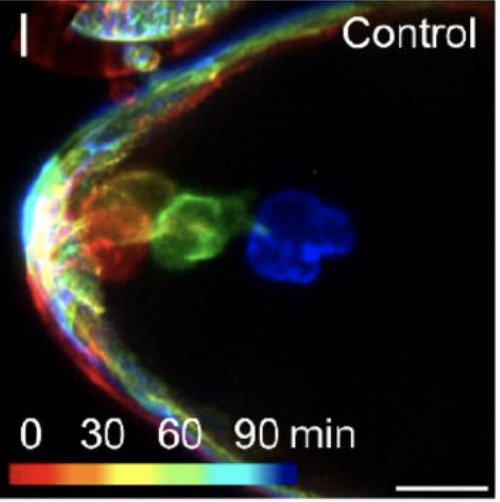
Septins Regulate Border Cell Shape and Surface Geometry ownstream of Rho | Developmental Cell
Gabbert, A., Mondo, J., Campanale, J., Mitchell, N., Myers, A., Streichan, S., Miolane, N., Montell, D.
Developmental Cell.
Septins self-assemble into polymers that bind and deform membranes in vitro and regulate diverse cell behaviors in vivo. How their in vitro properties relate to their in vivo functions is under active investigation. Here we uncover requirements for septins in detachment and motility of border cell clusters in the Drosophila ovary.
Read More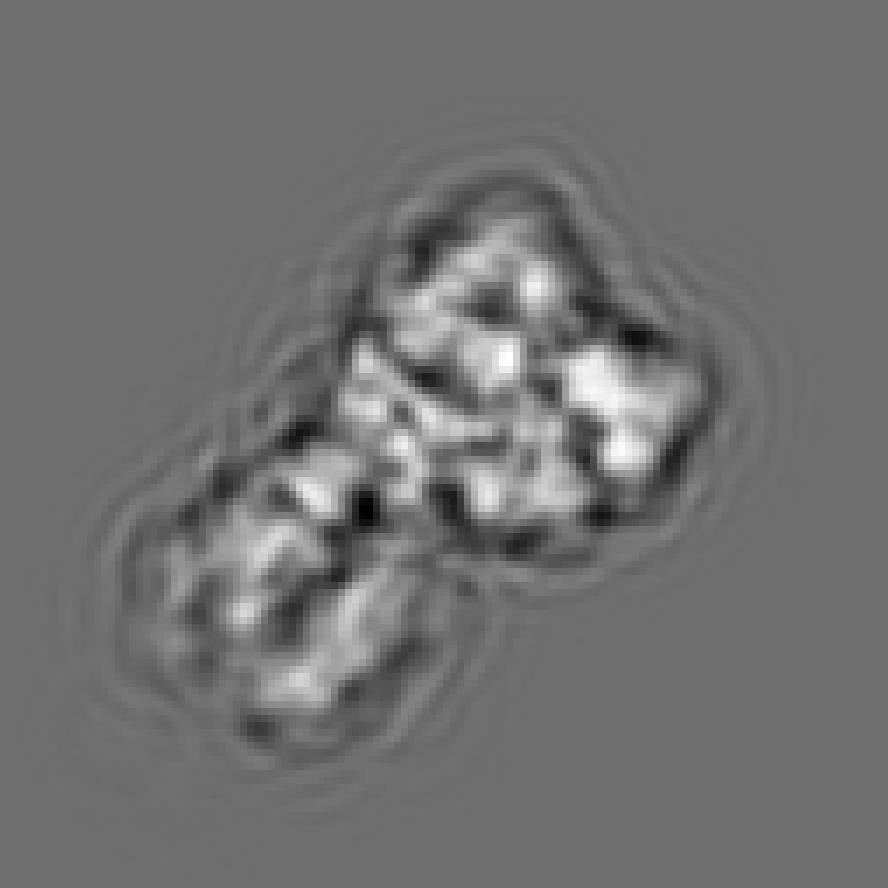
CryoChains: Heterogeneous Reconstruction of Molecular Assembly of Semi-flexible Chains from Cryo-EM Images | ICML CompBio Workshop
Koo, B., Martel, J., Peck, A., Levy, A., Poitevin, F., Miolane, N. [Paper]
We propose CryoChains to encode large deformations of biomolecules via rigid body transformation of their polymer instances (chains), while representing their finer shape variations with the normal mode analysis framework of biophysics. CryoChains gives a biophysically-grounded quantification of the heterogeneous conformations of biomolecules, while reconstructing their 3D molecular structures at an improved resolution compared to the current fastest, interpretable deep learning method.
Read More
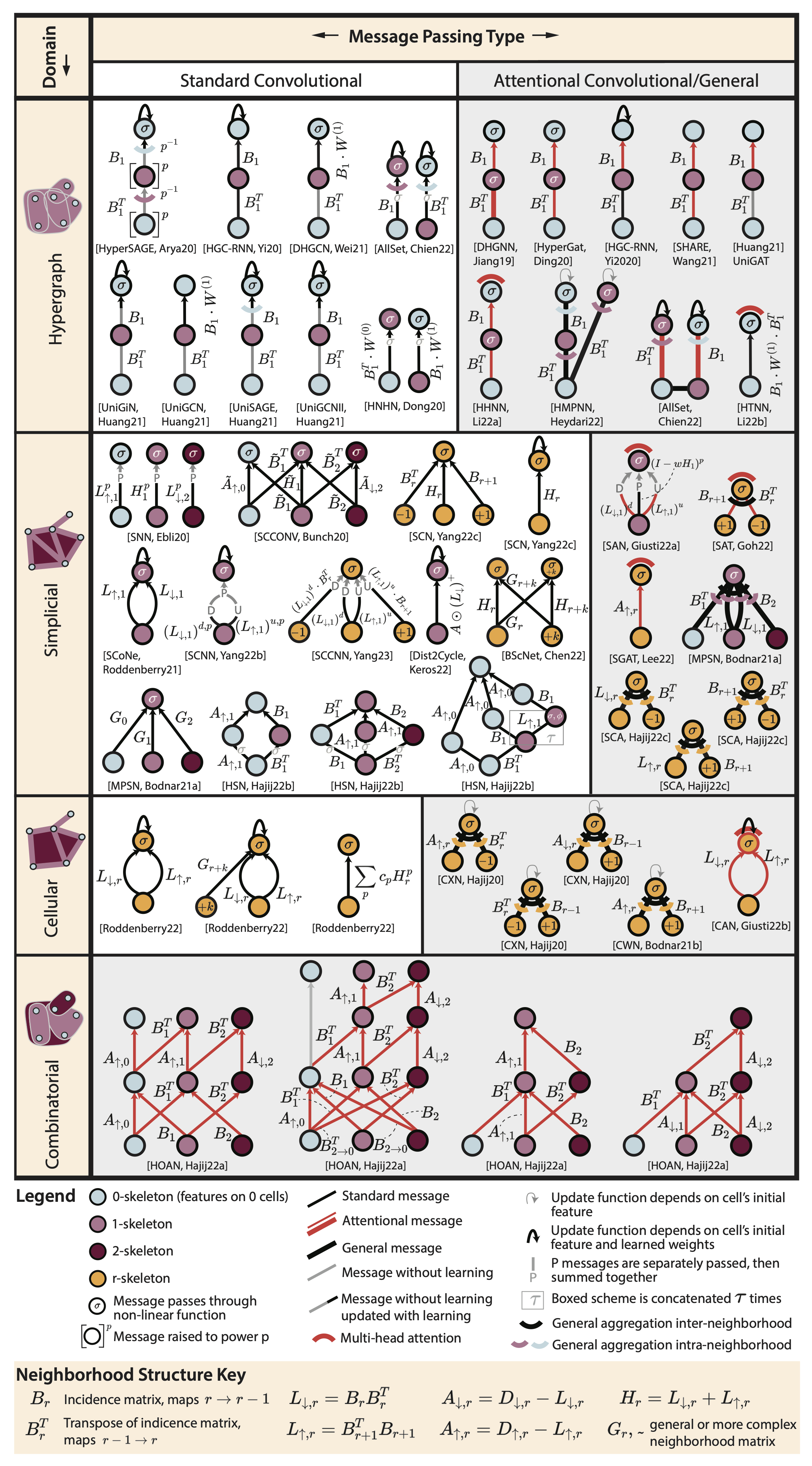
Architectures of Topological Deep Learning: A Survey on Topological Neural Networks | Submitted to PAMI: Transactions of Pattern Analysis and Machine Intelligence
Papillon, S., Sanborn, S., Hajij, M., Miolane, N. [Paper] [Equations]
Submitted to Nature Machine Intelligence.
Topological Neural Networks (TNNs) are deep learning architectures that process signals defined on topological domains. The domains of topological deep learning generalize the binary relations of graphs to hierarchical relations and higher-order set-based relations. The additional flexibility and expressivity of these architectures permits the representation of complex natural systems such as proteins, neural activity, and many-body physical systems. In this concise review of the latest in topological deep learning, we offer a pedagogical introduction to the field that uses a unified mathematical language to describe the landscape of TNNs.
Read More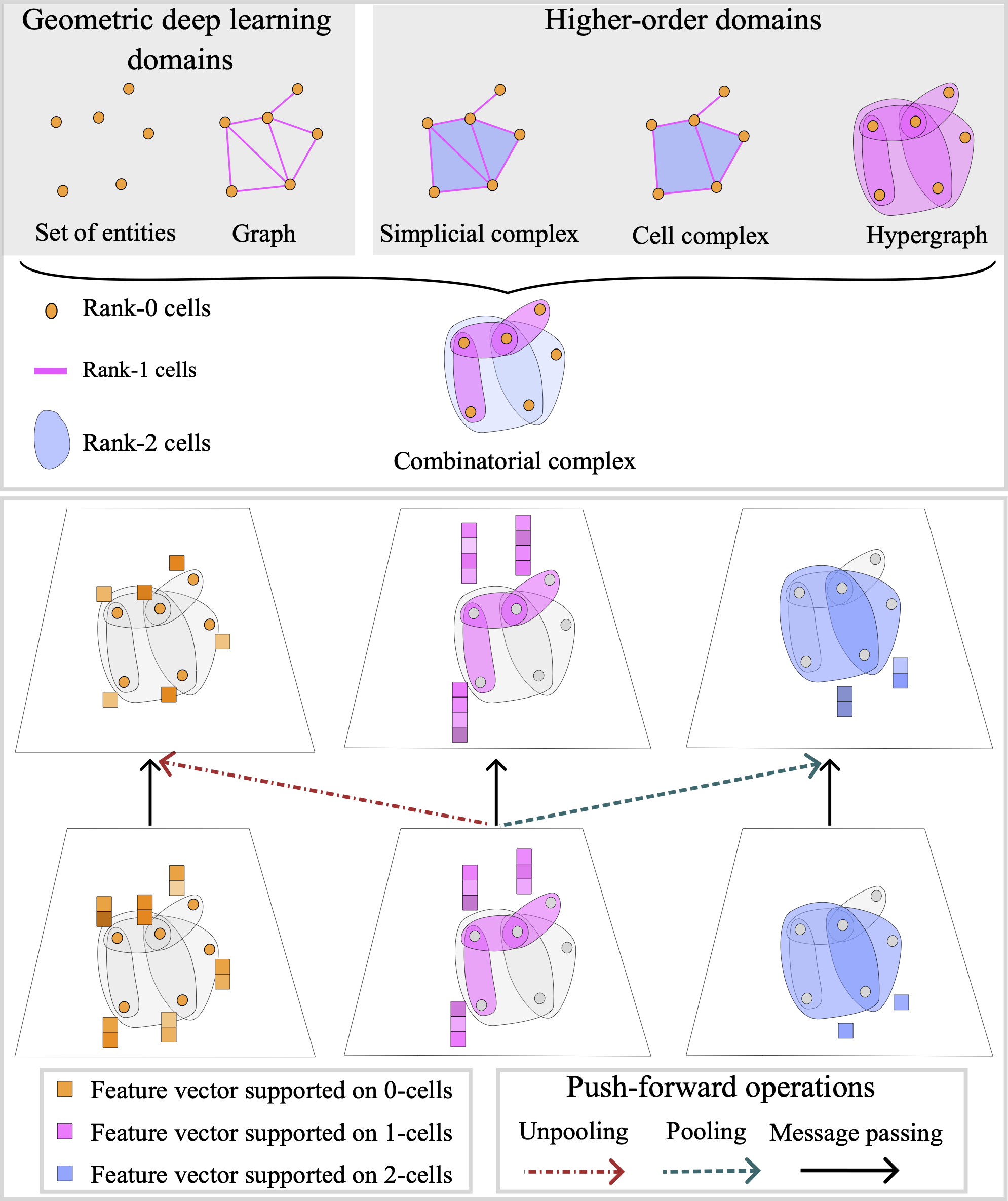
Topological Deep Learning: Going Beyond Graph Data | Submitted to JMLR: Journal of Machine Learning Research
Hajij, M., Zamzmi, G., Papamarkou, T., Miolane, N., Guzmán-Sáenz, A., Ramamurthy, K., Birdal, T., Dey, T., Mukherjee, S., Samaga, S., Livesay, N., Walters, R., Rosen, P., Schaub, M. [Paper] [Code]
Topological deep learning is a rapidly growing field that pertains to the development of deep learning models for data supported on topological domains such as simplicial complexes, cell complexes, and hypergraphs, which generalize many domains encountered in scientific computations. In this paper, we present a unifying deep learning framework built upon a richer data structure that includes widely adopted topological domains.
Read More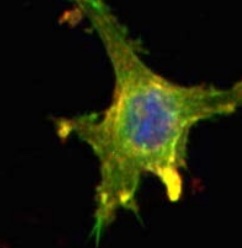
Orthogonal Outlier Detection and Dimension Estimation for Improved MDS Embedding of Biological Datasets | Frontiers in Bioinformatics
Li, W., Mirone, J., Prasad, A., Miolane, N., Legrand, C. and Dao Duc, K. [Paper]
Conventional dimensionality reduction methods are sensitive to orthogonal outliers, which yield significant defects in the embedding. We introduce a robust method to address this problem for cell morphology and human microbiomes datasets.
Read More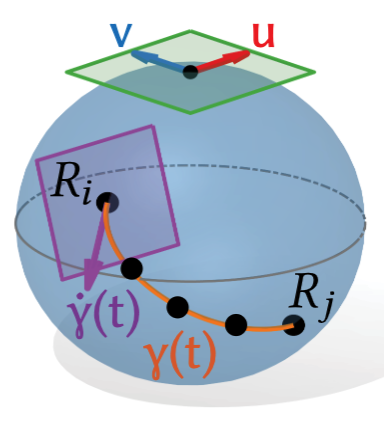
Interpolation of Animations on Lie Groups | Submitted
Kushner, S., Modi, V., Miolane, N.
Submitted to the International Conference on Computer Vision (ICCV).
We propose a family of Riemannian geodesic interpolation techniques to perform upsampling of low frame rate animations to high frame rates.
Read More
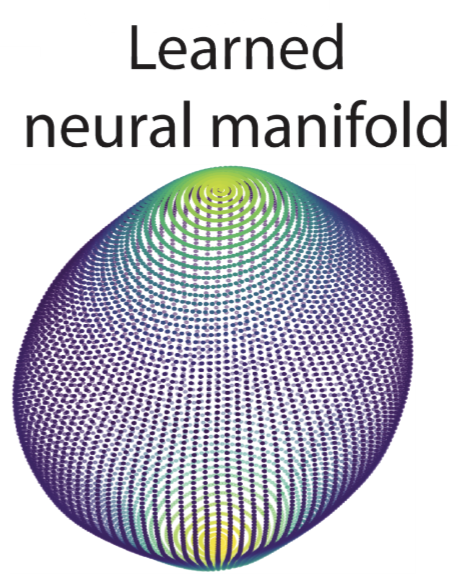
Quantifying Extrinsic Curvatures of Neural Manifolds | CVPRW
Acosta, F., Sanborn, S., Dao Duc, K., Madhav, M., Miolane, N.
CVPR Workshop Topology Algebra and Geometry (TAG) for Pattern Recognition with Applications.
We leverage tools from Riemannian geometry and topologically-aware deep generative models to introduce a novel approach for studying the geometry of neural manifolds. This approach (1) computes an explicit parameterization of the manifolds and (2) estimates their local extrinsic curvature. [Code].
Read More
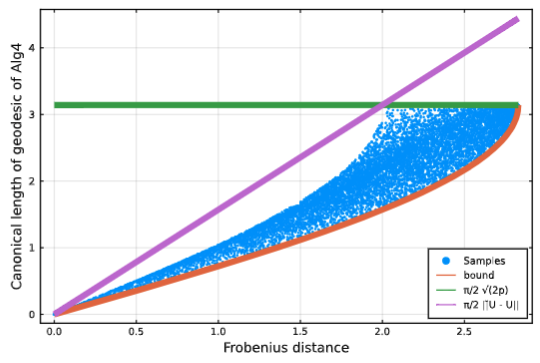
Lipschitz Constants Between Riemannian Metrics on the Stiefel Manifold | Geometric Science of Information
Mataigne, S., Absil, P.-A., Miolane, N. Geometric Science of Information.
We give the best Lipschitz constants between the distances induced by any two members of a one-parameter family of Riemannian metrics on the Stiefel manifold of orthonormal p-frames in n dimensions.
Read More
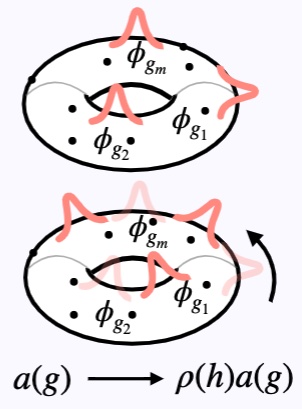
Equivariant Sparse Coding | Geometric Science of Information
Shewmake, C., Miolane, N., Olshausen, B. Geometric Science of Information. (Oral)
We describe a sparse coding model of visual cortex that encodes image transformations in an equivariant manner. We present results on time-varying visual scenes.
Read More
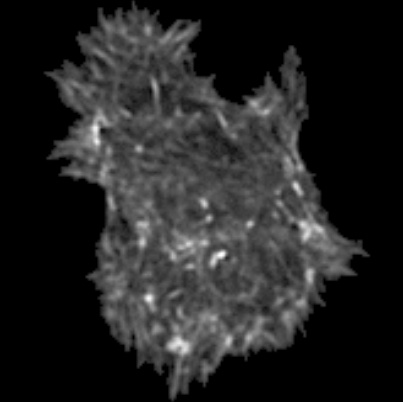
Using an Elastic Metric for Statistical Analysis of Tumor Cell Shape Heterogeneity | Geometric Science of Information
Li, W., Prasad, A., Miolane, N., Dao Duc, K. [Paper]. [Code]. Geometric Science of Information (GSI): Session Biological Shape Analysis. (Oral)
We propose a methodology grounded in geometric statistics to study and compare cellular morphologies from the contours they form on planar surfaces. We present findings on a dataset of images from osteocarcoma cells that includes different cancer treatments known to affect the cell morphology.
Read More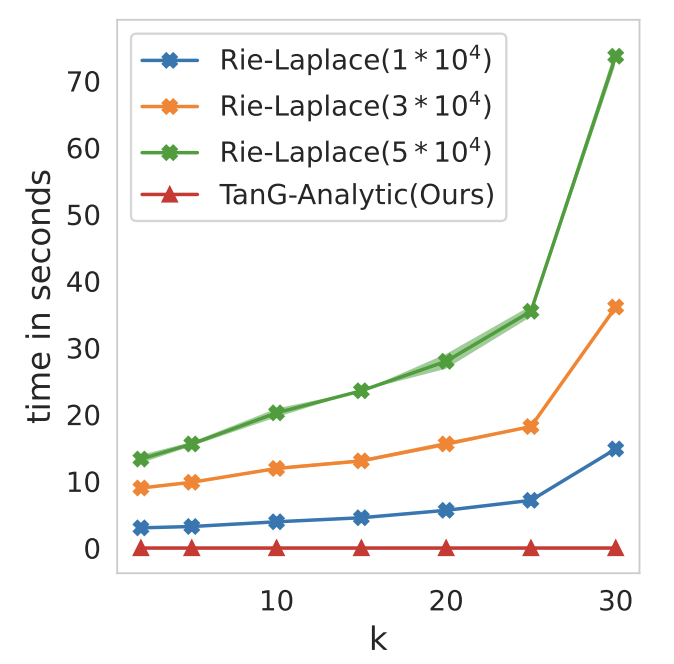
Differentially Private Fréchet Mean on the Manifold of Symmetric Positive Definite (SPD) Matrices | TMLR
Utpala, S., Vepakomma, P., Miolane, N.
Transactions of Machine Learning Research (TMLR).
We propose a novel, simple and fast mechanism - the Tangent Gaussian mechanism - to compute a differentially private Fréchet mean on the SPD manifold endowed with the log-Euclidean Riemannian metric. We show that our new mechanism obtains quadratic utility improvement in terms of data dimension over the current and only available baseline. Our mechanism is also simpler in practice as it does not require any expensive Markov Chain Monte Carlo (MCMC) sampling, and is computationally faster by multiple orders of magnitude - as confirmed by extensive experiments.
Read More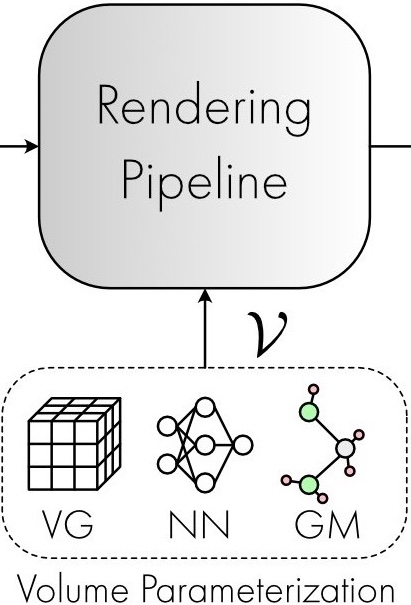
Deep Generative Modeling for Volume Reconstruction in Cryo-Electron Microscopy | JSB
Donnat, C., Levy, A., Poitevin, F., Zhong, E., Miolane, N.
Journal of Structural Biology.
Advances in cryo-electron microscopy (cryo-EM) for high-resolution imaging of biomolecules in solution have provided new challenges and opportunities. Next-generation volume reconstruction algorithms that combine generative modelling with end-to-end unsupervised deep learning techniques have shown promise, but many technical and theoretical hurdles remain. In light of the proliferation of such methods, we propose here a critical review of recent advances in the field of deep generative modelling for cryo-EM reconstruction. [Code].
Read More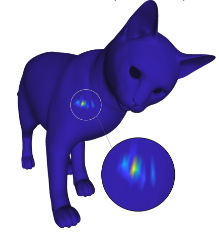
Probabilistic Riemannian Functional Map Synchronization for 3D Shape Correspondence | Submitted
Huq, F., Dey, A., Yusuf, S., Bazazian, D., Birdal, T., Miolane, N.
Submitted to Transactions of Machine Learning Research (TMLR).
We consider the problem of graph-matching on a network of 3D shapes with uncertainty quantification. We assume that the pairwise shape correspondences are efficiently represented as functional maps, that match real-valued functions defined over pairs of shapes. By modeling functional maps between nearly isometric shapes as elements of the Lie group SO(n), we employ synchronization to enforce cycle consistency of the collection of functional maps over the graph, hereby enhancing the accuracy of the individual maps.
Read More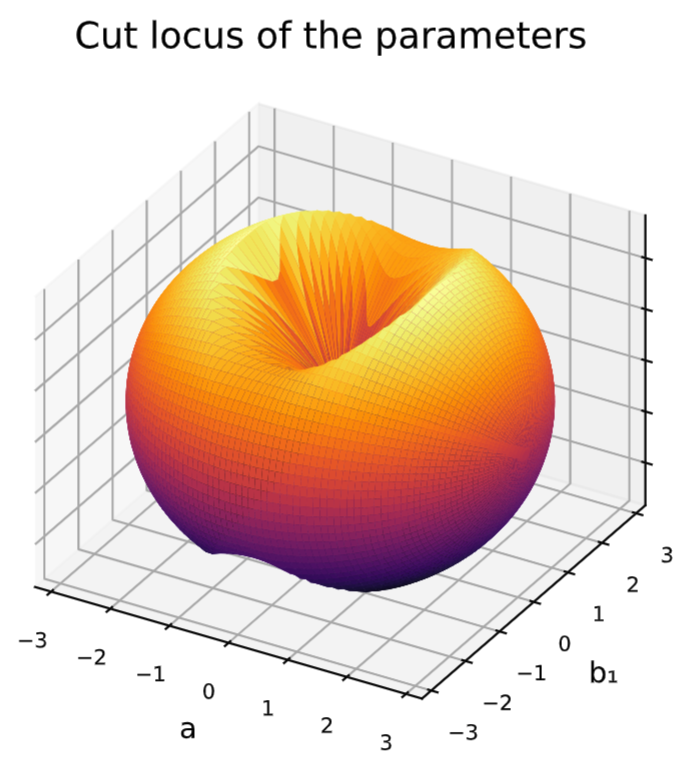
Computations on the Stiefel Manifold and the Special Orthogonal Group | In Prep.
Mataigne, S., Absil, P.-A., Miolane, N.
This master thesis presents new theoretical and computational developments of differential geometry on the Stiefel manifold of orthonormal p-frames in n-dimensions, and the Special Orthogonal group of rotations in n-dimensions.
Read More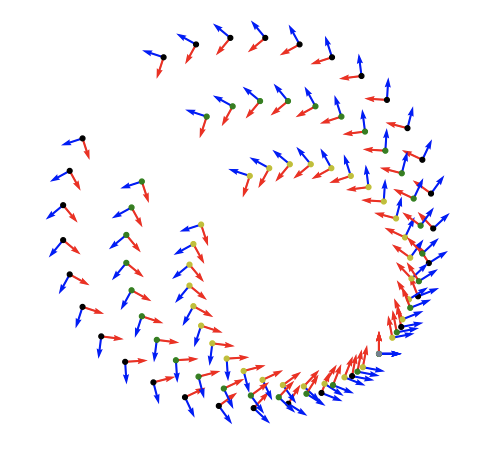
Introduction to Riemannian Geometry and Geometric Statistics: from theory to implementation with Geomstats | JFTML
Guigui, N., Miolane, N., Pennec, X..
Journal of Foundations and Trends in Machine Learning.
We give a self-contained exposition of the basic concepts of Riemannian geometry, providing illustrations and examples at each step and adopting a computational point of view. We cover the basics of differentiable manifolds, Riemannian manifolds, as well as quotient, homogeneous and symmetric spaces. Next, we demonstrate how these concepts are implemented in Geomstats, with details and code examples along the text. The culmination of this implementation is to be able to perform statistics and machine learning on manifolds, with as few lines of codes as in the wide-spread machine learning tool scikit-learn. [Code].
Read More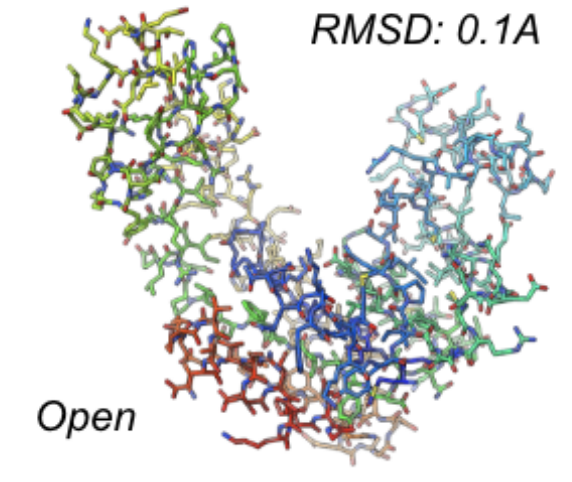
Heterogeneous Reconstructions of Deformable Models in Cryo-Electron Microscopy | NeurIPS MLSB
Nashed, Y., Peck, A., Martel, J., Levy, A., Koo, B., Wetzstein, G., Miolane, N., Ratner, D., Poitevin, F.
NeurIPS Workshop of Machine Learning for Structural Biology.
Cryogenic electron microscopy (cryo-EM) provides a unique opportunity to study the structural heterogeneity of biomolecules. Being able to explain this heterogeneity with atomic models would help our understanding of their functional mechanisms but the size and ruggedness of the structural space (the space of atomic 3D cartesian coordinates) presents an immense challenge. Here, we describe a heterogeneous reconstruction method based on an atomistic representation whose deformation is reduced to a handful of collective motions through normal mode analysis.
Read More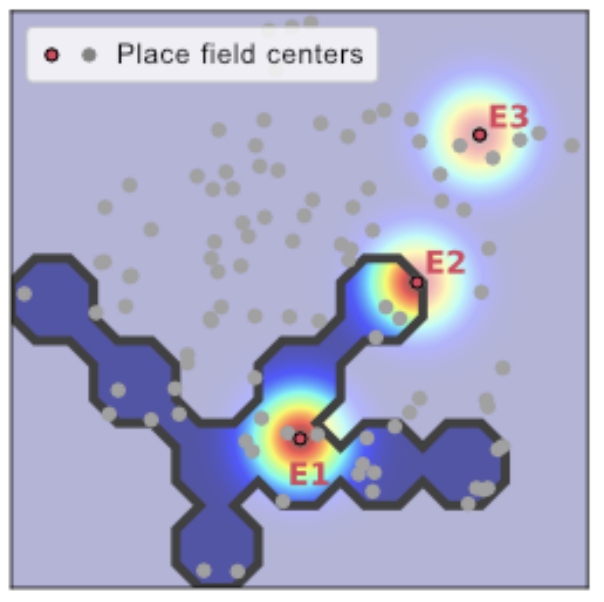
Testing Geometric Representation Hypotheses from Simulated Place Cells Recordings | NeurIPS NeurReps
Niederhauser, T., Lester, A., Miolane, N., Dao Duc, K., Madhav, M.
NeurIPS Workshop for Symmetry and Geometry in Neural Representations.
Hippocampal place cells can encode spatial locations of an animal in physical or task-relevant spaces. We simulated place cell populations that encoded either Euclidean- or graph-based positions of a rat navigating to goal nodes in a maze with a graph topology, and used manifold learning methods to analyze these neural population activities. [Code].
Read More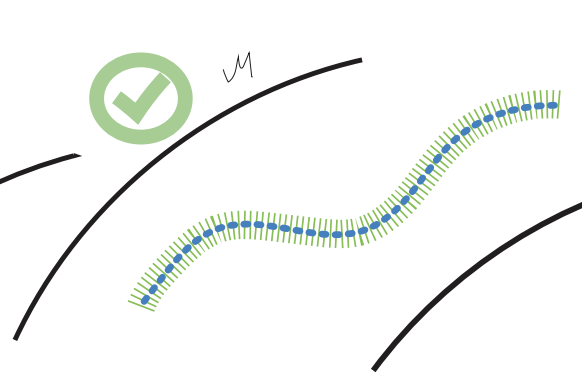
Regression-Based Elastic Metric Learning on Shape Spaces of Elastic Curves | NeurIPS LMRL
Myers, A., Miolane, N.
NeurIPS Workshop on Learning Meaningful Representations of Life.
We propose a metric learning paradigm, Regression-based Elastic Metric Learning (REML), which optimizes the elastic metric for geodesic regression on the manifold of discrete curves. Geodesic regression is most accurate when the chosen metric models the data trajectory close to a geodesic on the discrete curve manifold. When tested on cell shape trajectories, regression with REML's learned metric has better predictive power than with the conventionally used square-root-velocity (SRV) metric. [Code].
Read More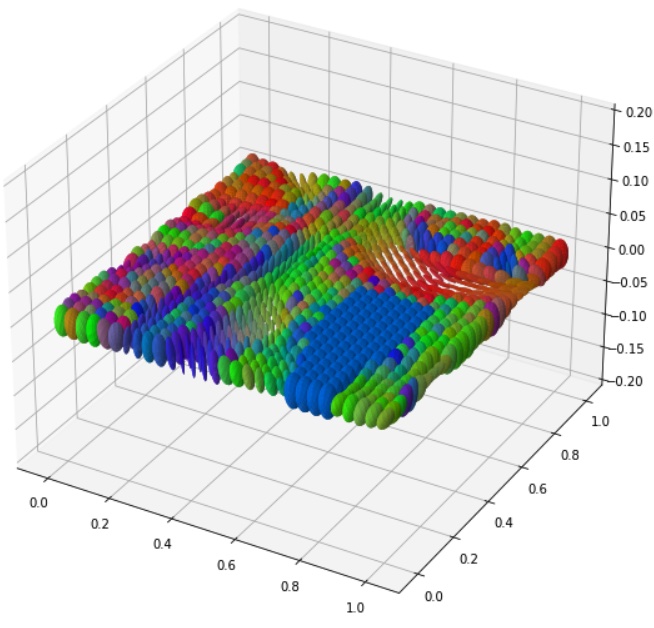
Challenge for Computational Geometry and Topology: Design and Results | ICLR GTRL
Myers, A., Utpala, ., Talbar, S., Sanborn, S., Shewmake, C., Donnat, C., Mathe, J., Lupo, U., Sonthalia, R., Cui, X., Szwagier, T., Pignet, A., Bergsson, A., Hauberg, S., Nielsen, D., Sommer, S., Klindt, D., Hermansen, E., Vaupel, M., Dunn, B., Xiong, J., Aharony, N., Pe'er, I., Ambellan, F., Hanik, M., Nava-Yazdani, E., von Tycowicz, C., Miolane, N.
ICLR Geometrical and Topological Representation Learning.
Proceedings of Machine Learning Research.
We present the computational challenge on differential geometry and topology that was hosted within the ICLR 2022 workshop "Geometric and Topological Representation Learning". The competition asked participants to provide implementations of machine learning algorithms on manifolds that would respect the API of the open-source software Geomstats (manifold part) and Scikit-Learn (machine learning part) or PyTorch. [Code].
Read More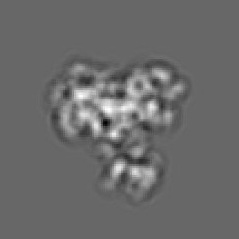
Defining an Action of SO(d)-Rotations on Projections of d-Dimensional Objects: Applications to Pose Inference with Geometric VAEs | GRETSI
Legendre, N, Dao Duc, K., Miolane, N.
GRETSI Conference (2022).
Recent advances in variational autoencoders (VAEs) have enabled learning latent manifolds as compact Lie groups, such as SO(d). Since this approach assumes that data lies on a subspace that is homeomorphic to the Lie group itself, we here investigate how this assumption holds in the context of images that are generated by projecting a d-dimensional volume with unknown pose in SO(d).
Read More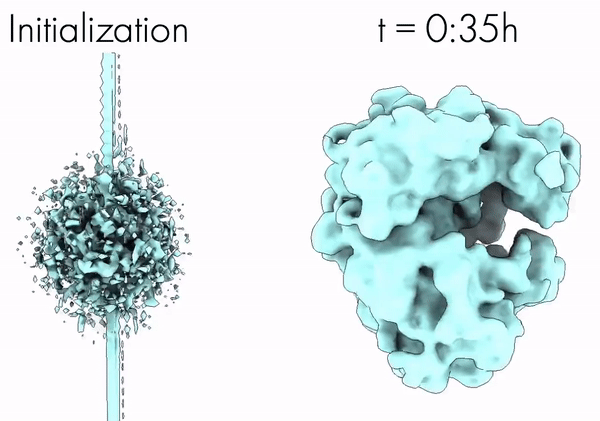
CryoAI: Amortized Inference of Poses for Ab Initio Reconstruction of 3D Molecular Volumes from Real Cryo-EM Images | ECCV
Levy, A., Poitevin, F., Martel, J., Nashed, Y., Peck, A., Miolane, N., Ratner, D., Dunne, M., Wetzstein, G.
4th International Symposium on Cryo-3D Image Analysis (Best Poster Award).
ECCV European Conference on Computer Vision.
Cryo-electron microscopy (cryo-EM) has become a tool of fundamental importance in structural biology. The algorithmic challenge of cryo-EM is to jointly estimate the unknown 3D poses and the 3D electron scattering potential of a biomolecule from millions of extremely noisy 2D images. Existing reconstruction algorithms, however, cannot easily keep pace with the rapidly growing size of cryo-EM datasets due to their high computational and memory cost. We introduce cryoAI, an ab initio reconstruction algorithm for homogeneous conformations that uses direct gradient-based optimization of particle poses and the electron scattering potential from single-particle cryo-EM data. [Code].
Read More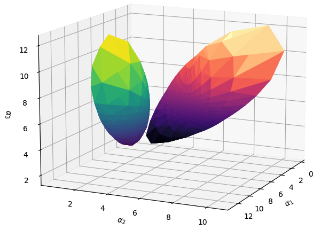
Parametric Information Geometry with the Package Geomstats | Transactions of Mathematical Software (TOMS)
Le Brigant, A., Deschamps, J., Collas, A., Miolane, N.
We introduce the information geometry module of the Python package Geomstats. The module first implements Fisher-Rao Riemannian manifolds of widely used parametric families of probability distributions, such as normal, gamma, beta, Dirichlet distributions, and more. The module further gives the Fisher-Rao Riemannian geometry of any parametric family of distributions of interest, given a parameterized probability density function as input. [Code].
Read More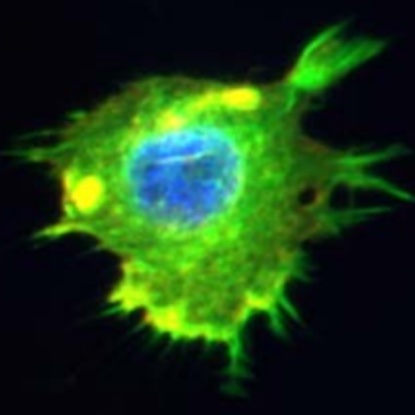
Biological Shape Analysis with Geometric Statistics and Learning | Oberwolfach Snapshots
Utpala, S., Miolane, N.
Oberwolfach Snapshots of Modern Mathematics (2022).
The advances in biomedical imaging techniques have enabled us to access the 3D shapes of a variety of structures: organs, cells, proteins. Since biological shapes are related to physiological functions, shape data may hold the key to unlocking outstanding mysteries in biomedicine. This snapshot introduces the mathematical framework of geometric statistics and learning and its applications in biomedicine.
Read More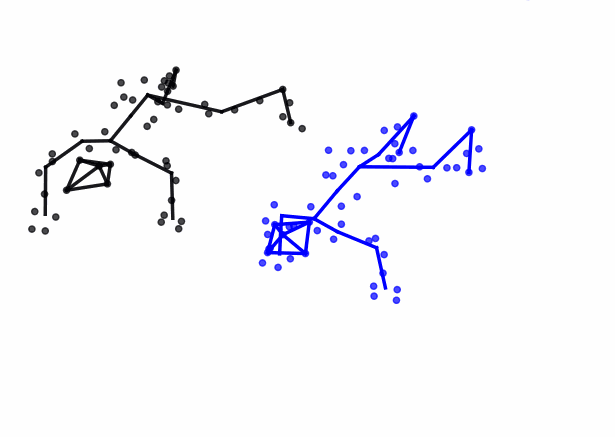
Intentional Choreography with Semi-Supervised Recurrent Variational Autoencoders | NeurIPS CAD
Papillon, M., Pettee, M., Miolane, N.
NeurIPS Workshop of Creativity and Design.
Given a small amount of dance sequences labeled with qualitative choreographic annotations, PirouNet conditionally generates dance sequences in the artistic style of the choreographer. [Code].
Read More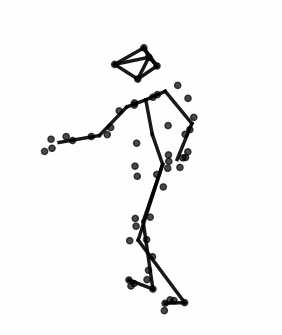
PirouNet: Creating Dance through Artist-Centric Deep Learning | EAI ArtsIT
Papillon M., Pettee M., Miolane N.
EAI ArtsIT Conference. Best Paper Award (Oral).
Using Artificial Intelligence (AI) to create dance choreography with intention is still at an early stage. Methods that conditionally generate dance sequences remain limited in their ability to follow choreographer-specific creative direction, often relying on external prompts or supervised learning. In the same vein, fully annotated dance datasets are rare and labor intensive. To fill this gap and help leverage deep learning as a meaningful tool for choreographers, we propose "PirouNet": PirouNet allows dance professionals to annotate data with their own subjective creative labels and subsequently generate new bouts of choreography based on their aesthetic criteria. Thanks to the proposed semi-supervised approach, PirouNet only requires a small portion of the dataset to be labeled, typically on the order of 1%.. [Code].
Read More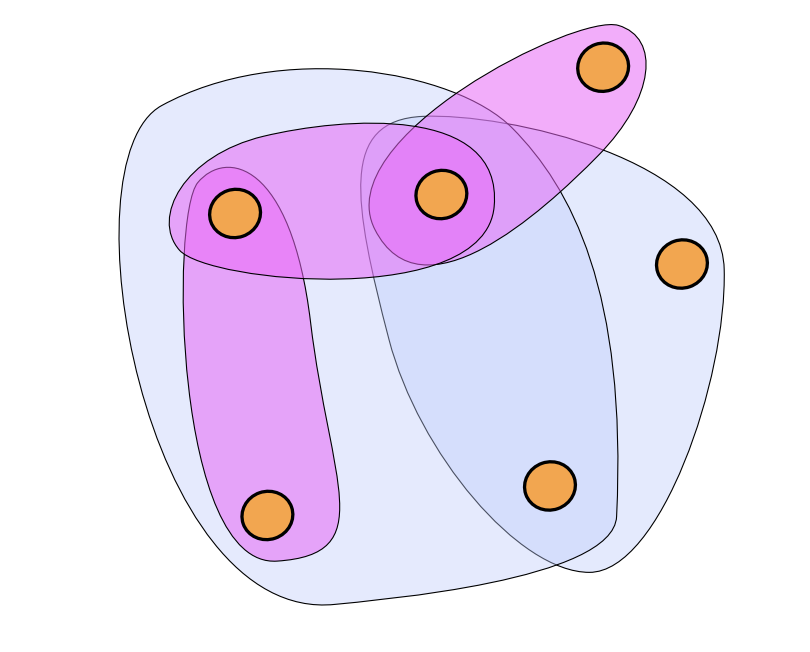
Higher-Order Attention Networks | ArXiv
Hajij, M., Zamzmi, G., Papamarkou, T., Miolane, N., Guzman-Saenz, A., Ramamurthy, K., N.
We introduce higher-order attention networks (HOANs), a novel class of attention-based neural networks defined on a generalized higher-order domain called a combinatorial complex (CC). Similar to hypergraphs, CCs admit arbitrary set-like relations between a collection of abstract entities. Simultaneously, CCs permit the construction of hierarchical higher-order relations analogous to those supported by cell complexes. Thus, CCs effectively generalize both hypergraphs and cell complexes and combine their desirable characteristics.
Read More
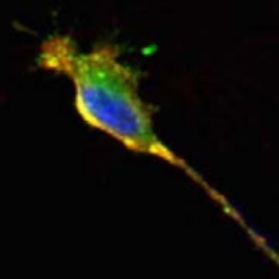
Challenge for Computational Geometry & Topology: Design and Results | ICLR GTRL
Miolane, N., Caorsi, M., Lupo, U., Guerard, M., Guigui, N., Mathe, J., Cabanes, Y., Reise, W., Davies, T., Leitão, A., Mohapatra, S., Utpala, S., Shailja, S., Corso, G., Liu, G., Iuricich, F., Manolache, A., Nistor, M., Bejan, M., Mihai Nicolicioiu, A., Luchian, B.-A., Stupariu, M.-S., Michel, F., Dao Duc, K., Abdulrahman, B., Beketov, M., Maignant, E., Liu, Z., Černý, M., Bauw, M., Velasco-Forero, S., Angulo, J., Long Y.
ICLR Workshop on Geometrical and Topologic Representation Learning.
We present the computational challenge on differential geometry and topology that happened within the ICLR 2021 workshop "Geometric and Topological Representation Learning". The competition asked participants to provide creative contributions to the fields of computational geometry and topology through the open-source repositories Geomstats and Giotto-TDA. [Code].
Read More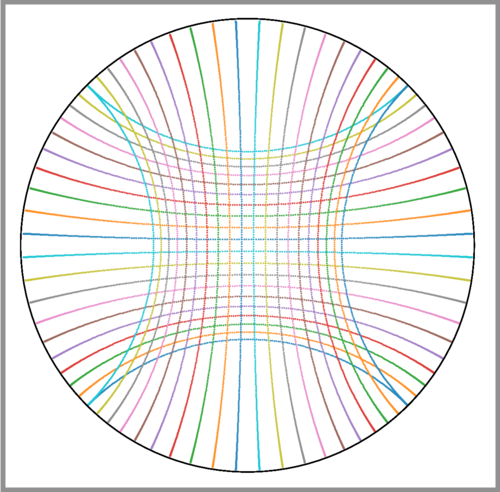
Geomstats: A Python Package for Riemannian Geometry in Machine Learning | JMLR
Miolane, N., Guigui, N., Le Brigant, A., Mathe, J., Hou, B., Thanwerdas, Y., Heyder, S., Peltre, O., Koep, N., Cabanes, Y., Chauchat, P., Zaatiti, H., Hajri, H., Gerald, T. , Shewmake, C., Brooks, D., Kainz, B., Donnat, C., Holmes, S., Pennec, X.
Journal of Machine Learning Research.
We introduce Geomstats, an open-source Python toolbox for computations and statistics on nonlinear manifolds, such as hyperbolic spaces, spaces of symmetric positive definite matrices, Lie groups of transformations, and many more. [Code].
Read More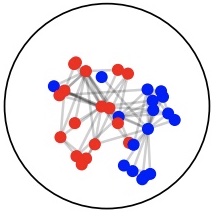
Introduction to Geometric Learning in Python with Geomstats | SciPy
Miolane, N., Guigui, N., Zaatiti, H., Shewmake, C., Hajri, H., Brooks, D., Le Brigant, A., Mathe, J. Hou, B., Thanwerdas, Y., Heyder, S., Peltre, O., Koep, N., Cabanes, Y., Gerald, T. Chauchat, P., Kainz, B., Donnat, C., Holmes, S., Pennec, X.
SciPy Conference on Scientific Computing in Python.
There is a growing interest in leveraging differential geometry in the machine learning community. Yet, the adoption of the associated geometric computations has been inhibited by the lack of a reference implementation. To address this gap, we present the open-source Python package geomstats and introduce hands-on tutorials for differential geometry and geometric machine learning algorithms. [Code].
Read More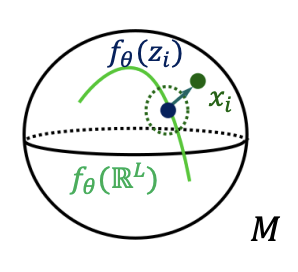
Learning Weighted Submanifolds With Riemannian Variational Autoencoders | CVPR
Miolane, N., Holmes, S.
CVPR Conference of Computer Vision and Pattern Recognition.
Manifold-valued data naturally arises in medical imaging. One of the challenges that naturally arises consists of finding a lower-dimensional subspace for representing such manifold-valued data. Traditional techniques, like principal component analysis, are ill-adapted to tackle non-Euclidean spaces. We introduce Riemannian Variational Autoencoders to perform weighted submanifold learning powered by amortized variational inference.
Read More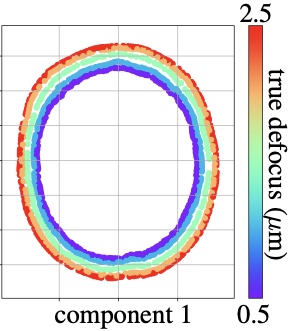
Estimation of Orientation and Camera Parameters from Cryo-Electron Microscopy Images with Variational Autoencoders and Generative Adversarial Networks | CVPRW
Miolane, N., Poitevin, F., Li, Y.-T., Holmes, S.
CVPR Workshop on Computer Vision for Microscopy Imaging.
We combine variational autoencoders (VAEs) and generative adversarial networks (GANs) to learn a low-dimensional latent representation of cryo-EM images. Cryo-electron microscopy (cryo-EM) is capable of producing reconstructed 3D images of biomolecules at near-atomic resolution. As such, it represents one of the most promising imaging techniques in structural biology.
Read More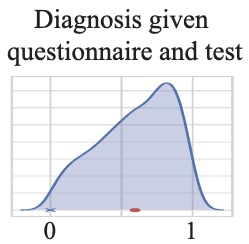
A Bayesian Hierarchical Network for Combining Heterogeneous Data Sources in Medical Diagnoses | NeurIPS ML4H
Donnat, C., Miolane, N., Bunbury, F., Kreindler, J.
NeurIPS Workshop on Machine Learning for Health.
1st Prize: C3.ai Grand Covid Challenge (100,000$).
Computer-Aided Diagnosis has shown stellar performance in providing accurate medical diagnoses across multiple testing modalities (medical images, electrophysiological signals, etc.). While this field has typically focused on fully harvesting the signal provided by a single (and generally extremely reliable) modality, fewer efforts have utilized imprecise data lacking reliable ground truth labels. We devise a Stochastic Expectation-Maximization algorithm that allows the principled integration of heterogeneous, and potentially unreliable, data types. We showcase the practicality of this approach by deploying it on a real COVID-19 immunity study.
Read MoreBias on Estimation in Quotient Space and Correction Methods | Elsevier
Miolane, N., Devilliers, L., Pennec, X.
Chapter in Riemannian Geometric Statistics in Medical Imaging. Statistics on Shape Spaces. Elsevier.
Read MoreExploring Cryo-EM Latent Space with Variational Autoencoders | Stanford Bio-X
Miolane, N., Poitevin, F., Holmes, S.
Stanford Bio-X Workshop on Cryo-Electron Microscopy.
Read MoreConvenience Tools to Explore Variability in Cryo-EM Data | Stanford Bio-X
Poitevin, F., Li, Y.T., Miolane, N., Gati, C., Levitt, M.
Stanford Bio-X Workshop on Cryo-Electron Microscopy.
Read MorePVNet: A LRCN Architecture for Spatio-Temporal Photovoltaic Power Forecasting from Numerical Weather Prediction | ICML AICC
Mathe, J., Miolane, N., Sebastien, N., Lequeux, J.
ICML Workshop on AI for Climate Change.
Read MoreComputing CNN Loss and Gradients for Pose Estimation with Riemannian Geometry | MICCAI
Hou, B., Miolane N., Khanal B., Lee M., Alansary A., McDonagh S., Hajnal J., Rueckert D., Glocker B., Kainz B.
MICCAI Conference on Medical Image Computing and Computer Assisted Intervention.
Read MoreTopologically Constrained Template Estimation | SIAGA
Miolane, N., Holmes, S., Pennec, X.
SIAM Journal on Applied Algebra and Geometry.
Read MoreTemplate Shape Estimation in Computational Anatomy | SIIMS
Miolane, N., Holmes, S., Pennec, X.
SIAM Journal of Imaging Science.
Read MoreToward a Unified Geometric Bayesian Framework for Template Estimation in Computational Anatomy. | ISBA
Miolane, N., Pennec, X., Holmes, S.
ISBA World Meeting of the International Society for Bayesian Analysis. (Young Researcher Travel Award).
Read MoreAnalyse Biométrique de l'Anneau Pelvien en 3 Dimensions | JRCOT
Darmante, H., Bugnas, B., Dompsure, R.B.D., Barresi, L., Miolane, N., Pennec, X., de Peretti, F., Bronsard, N.
Journal Revue de Chirurgie Orthopédique et Traumatologique.
Read MoreStatistics on Lie Groups: Can We Obtain a Consistent Framework with Pseudo-Riemannian Metrics? | GMV
Miolane, N.
Institut Henri Poincaré Workshop on Geometrical Models in Vision.
Read More